Our Blog
STAY INFORMED
When Will AI Surpass Human Intelligence?
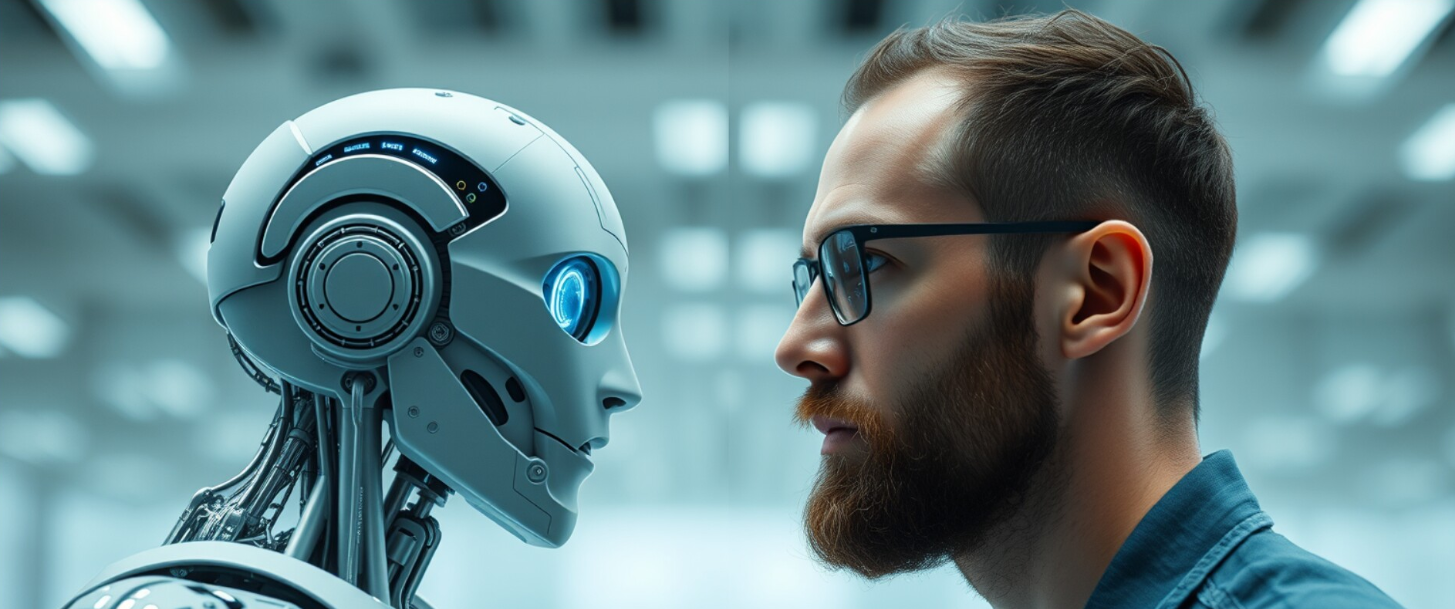
This question often reflects a misunderstanding of AI and its evolution. In Our Workshops and Training Sessions, we explain to the participants that what was once called "AI" often becomes routine over time. For example, in 1968, the A* algorithm (used to find the shortest path between two points) was considered cutting-edge AI. Today, we use far more advanced algorithms in navigation apps, but no one thinks of them as "AI".
Now, Large Language Models (LLMs) like ChatGPT are the new face of AI. But what are LLMs? In short, they're trained models that generate text based on the input you provide, known as a "prompt." Despite their impressive abilities, they aren't sentient or capable of self-learning, which explains why they struggle with tasks like counting letters in a word or solving simple math problems without additional tricks.
What made ChatGPT possible?
It all started with a 2017 paper published by Google researchers, which introduced the Transformer architecture. This breakthrough allowed AI to process language more effectively by focusing on certain words more than others to retain context, a game-changer for natural language processing. It also paved the way for other models capable of generating images, music, and even video from natural language prompts.
GPT (Generative Pre-Trained Transformer) models have since evolved, with GPT-3 containing 175 billion parameters.
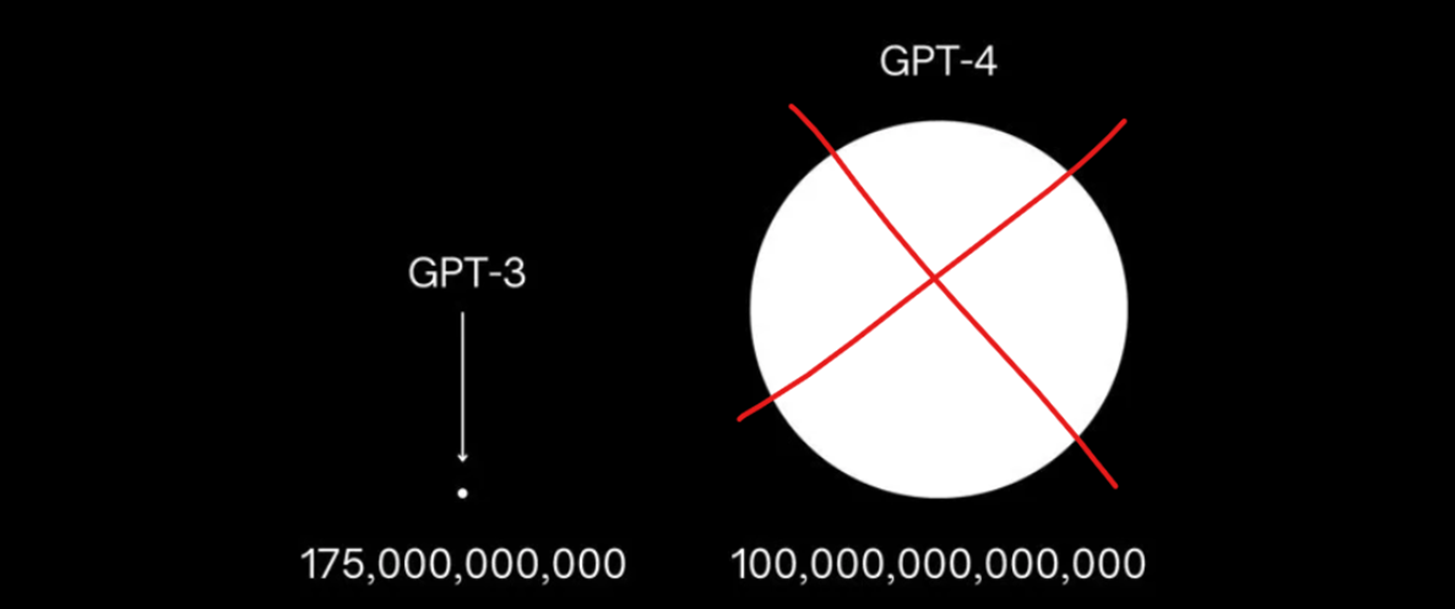
We’ve seen these sensational graphs circulated in early 2023, claiming that GPT-4 would have 100 trillion parameters, a massive leap from GPT-3. These assertions were quickly debunked, even by OpenAI's CEO Sam Altman. But why do we still see these graphs circulating? The reason is that many companies benefit from this inflated hype, but soon reality hits.
More parameters doesn't mean more intelligence
As our expectations grow, the challenge isn't just adding more parameters, machine learning researchers have already pushed the limits of what we can do with available data and current hardware at scale. The trend now is not increasing the size of one model or further training on more data; instead we use multiple specialized models to improve the overall quality of the output, like OpenAI’s recent o1-preview, which uses several models behind the scenes to boost the accuracy of chatGPT output.
How to reach true human-like AI or AGI?
According to AI researcher Yann LeCun, the answer isn't just better hardware or software. Humans learn through rich, multisensory experiences, not just text and language. Until AI can similarly interact with the physical world, AGI remains out of reach.